Trung Q. Duong
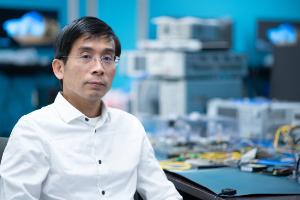
Canada Excellence Research Chair
Full Professor at Memorial University of Newfoundland, Canada
He is a Canada Excellence Research Chair and a Full Professor at Memorial University of Newfoundland, Canada. His current research focuses on quantum machine learning, signal processing, real-time optimization, quantum optimization, 5G/6G wireless communications, Internet-of-Things (applied to healthcare, disaster management, energy, agriculture, environment, and smart cities). Currently, his main research interests in 5G/6G include:
- Joint optimal design for communications and computing
- Digital twin networks
- Integrated satellite and terrestrial networks
- Quantum machine learning for dynamic radio resource allocation in wireless networks
- Real-time optimisation for unmanned aerial vehicles (UAV)
- Semantic communications
- Integrated sensing and communications
- Physical layer security
He has established a strong record in these areas with 500+ publications (Google Scholar: 20,000+ citations, h-index of 76). He was the only UK-based researcher to have received both prestigious awards from the Royal Academy of Engineering: i) the Royal Academy of Engineering Research Fellowship (2015–2020) and ii) Research Chair of Royal Academy of Engineering (2020-2025). He has received $54+ million in research grants from Canada NSERC, EU Horizon, UK funding bodies (EPSRC, ESRC, Innovate UK, Royal Academy of Engineering, Royal Society, DfE, British Council), international funding bodies, and industry. He has served as an Editor for major technical journals including IEEE Trans. on Wireless Communications, IEEE Trans. on Communications, IEEE Trans. on Vehicular Technology, IEEE Wireless Communications Letters, IEEE Communications Letters, and IEEE Communications Surveys & Tutorials. He is an IEEE Fellow, AAIA Fellow, and a recipient of the prestigious Newton Prize awarded in 2017 by the UK Government.
Title
Hybrid Quantum-Classical Learning and Optimization for 6G Networks